The population of large cities is increasingly gravitating toward urban centers, bringing both challenges and opportunities. Among the most pressing issues is traffic congestion, a problem that demands urgent solutions as urban density continues to rise.
What steps are being taken to ensure that population growth does not negatively impact the environment? One promising approach lies in Artificial Intelligence (AI), which extends far beyond architecture and urban planning. AI could be the key to improving city traffic management, optimizing public transportation, and making urban areas more efficient and sustainable.
At the same time, Big Data has long been a critical tool for transportation agencies seeking to analyze and address traffic issues. But now, AI is playing an increasingly important role in predicting traffic patterns and outcomes, allowing cities to implement proactive rather than reactive solutions.
Introduction to the Challenges of Urban Traffic
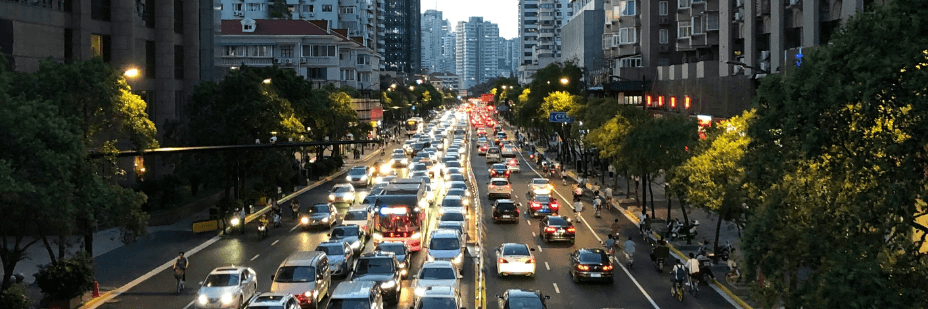
As urban populations continue to grow, traffic congestion has become an increasingly urgent issue. A first analysis of the problem can be divided into three key areas:
- Increasing traffic density: Urban areas are becoming more crowded, leading to higher volumes of vehicles on the roads.
- Inefficient public transport: Many transit systems are not adequately structured to meet the growing demands of urban populations, resulting in overcrowding and service delays.
- Rising pollution levels: Increased traffic leads to higher emissions, which contribute to environmental and public health concerns. According to a Yale study, prolonged exposure to pollutants regulated by the EPA—excluding carbon monoxide and lead—can cause airway hyperresponsiveness. Over time, this exposure may increase respiratory infections, exacerbate symptoms, and impair lung function, particularly in children.
Adding to these challenges, the lack of real-time Big Data analytics makes it difficult for cities to implement effective traffic management plans and respond promptly to changing conditions.
The Role of Big Data in Traffic Management
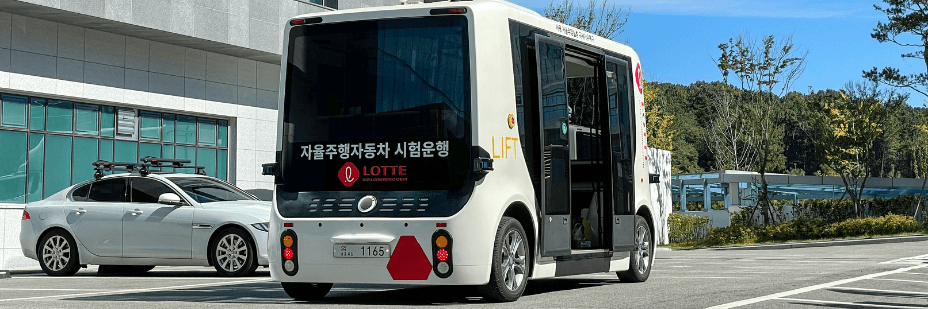
The growing availability of input, combined with the synergy between Artificial Intelligence (AI) and Big Data, is playing a crucial role in the development of intelligent traffic systems. These technologies optimize traffic planning, forecasting, and management, reducing transportation costs while improving performance across public transit, ride-sharing, and smart parking sectors.
Systems leveraging AI and Big Data can enhance urban environments by providing real-time adjustments and predictive analysis. This is achieved through the integration of IoT devices, sensors, and connected infrastructure, enabling data-driven decision-making that fosters innovation, investment, and sustainable urban development.
Looking ahead, this technological evolution is paving the way for Connected and Automated Mobility (CAM)—a future where autonomous and connected vehicles operate without human intervention. By advancing safety standards and expanding shared mobility services, CAM has the potential to further optimize urban traffic management and overall efficiency.
Applications of Artificial Intelligence in Traffic Control and Optimization
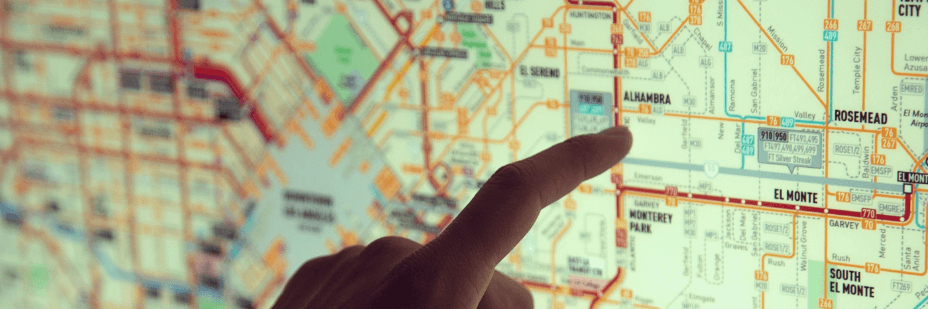
AI-driven traffic management systems leverage both historical and real-time data to conduct precise predictive analyses, allowing authorities to efficiently manage, redirect, and optimize traffic flow. By continuously monitoring traffic patterns, these systems provide proactive solutions to prevent congestion.
Singapore’s Land Transport Authority (LTA) has implemented machine learning to predict traffic conditions and dynamically modify routes. This system collects real-time data from GPS-equipped vehicles, traffic cameras, and even social media, offering drivers alternative, less congested routes.
Smart, AI-powered traffic lights analyze data from sensors, cameras, and GPS devices, enabling adaptive traffic control. By continuously adjusting signal timing based on real-time conditions, they improve traffic efficiency. An example is San Diego’s Smart Traffic Control system, which uses Big Data from connected vehicles to optimize traffic light coordination.
In public transportation, AI already assists in determining the most efficient routes, schedules, and transport capacities, while also reporting disruptions and accidents in real-time. It enhances safety monitoring by detecting passengers standing too close to platform edges, identifying individuals in distress, and allowing swift staff intervention. AI systems also aid in detecting fare evasion, abandoned objects, weapons, and other potential security threats.
Additionally, AI is being explored to enhance urban cycling infrastructure. Transport for London (TfL) is using AI-driven analytics to assess and improve cycling lane utilization, encouraging sustainable mobility solutions.
Challenges and Limitations

While Artificial Intelligence and Big Data offer transformative potential for traffic management, several unresolved challenges and concerns remain.
One major issue is ethics. These technologies are designed to save and improve lives, but in extreme cases, AI-driven systems might face difficult moral decisions. For example, how would an autonomous vehicle decide between two unavoidable collisions? The ethical framework guiding these decisions remains a critical concern.
Another significant risk is cybersecurity. AI-powered, connected vehicles and traffic control systems are vulnerable to hacking. A compromised system could allow unauthorized control over vehicles or infrastructure, posing serious threats to public safety. Additionally, data privacy breaches could expose sensitive passenger information, disrupt transport networks, and erode public trust in AI-driven mobility solutions.
Lastly, high costs remain a barrier. Implementing AI-based traffic management requires substantial investment, which could disadvantage economically weaker regions. Developing nations may struggle to afford these advanced systems, leading to a widening technological gap in urban mobility.
Future Trends in Intelligent Traffic Management
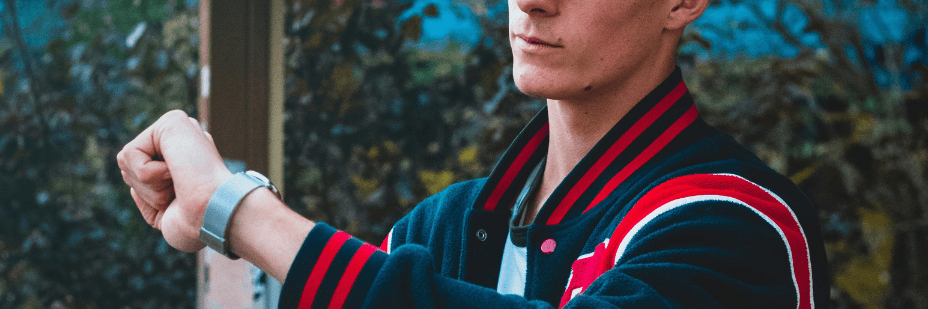
Despite these challenges, AI-driven mobility solutions are rapidly expanding. A McKinsey report predicts that integrating AI into urban transport could reduce commuting time by 20%, increase on-time arrivals by 10%, and cut passenger waiting times by 15%.
Looking ahead, the integration of 5G technology with AI is expected to revolutionize urban mobility. The high-speed, low-latency capabilities of 5G will enable real-time traffic monitoring, allowing for instant decision-making in complex urban environments.
Additionally, AI-driven traffic optimization will reduce fuel consumption and minimize greenhouse gas emissions, tackling pollution and lowering the risk of respiratory diseases. By ensuring smoother traffic flow, AI can contribute to more sustainable and efficient urban transportation networks.